Data Quality: It’s More Important Than you Think
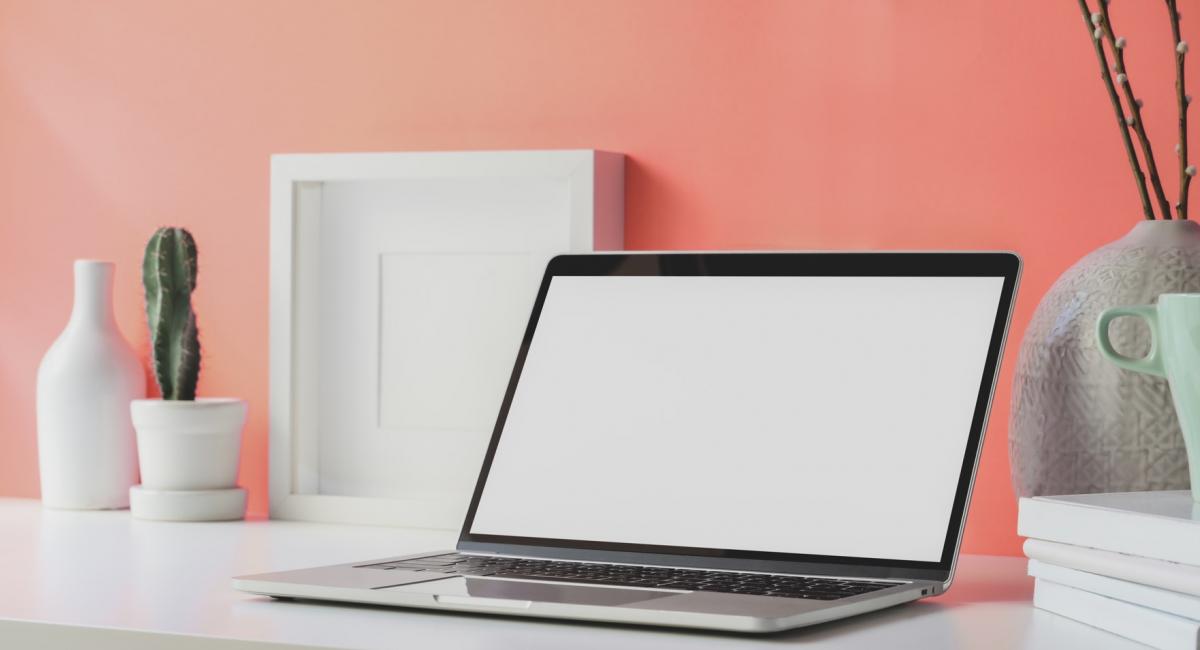
I’ll tell you a secret. The key to a successful data project – whether it’s an integration, migration, AI initiative, data lake, big data project, or otherwise — is data quality.
Ok, it’s not exactly a “secret”, but even as IT professionals shout this mantra from the rooftops, many organizations continue their struggle to maintain quality data. Poor data quality can be the result of:
ZoomInfo recently reported that 94% of businesses suspect their customer and prospect data is inaccurate, and an estimated 40% of their business objectives fail due to poor data quality. On the flip side, organizations with clean data generate up to 70% more revenue than their dirty data counterparts.
So, if this is true, why are so many businesses still failing to adopt proper data quality?
One of the biggest inhibitors is the perceived cost. Quality data requires ongoing efforts beyond a single data cleaning event. Many organizations are afraid of what an investment like that may look like. Unfortunately, the longer a company waits to reign in data quality, the more expensive it becomes. Bad data costs businesses more than $611 billion every year.
Data quality misconceptions can blind you to the importance of a full data quality approach. We’ll touch on those myths next, and then I’ll offer some tips for how to improve your data quality.
Optimizing your data requires more than a single action, approach, or departmental initiative. Data quality is an ongoing organization-wide process. It must be approached thoughtfully and with a comprehensive plan. Once you set that plan in motion, maintaining those standards should be easier, but you must first overcome your organization’s own misconceptions about data quality. Here are some of the most common myths:
Data cleaning is an extremely important aspect of data quality, but it’s not the entire picture. How the data is being used across the organization can also impact its quality. Even if the data itself is accurate, if pieces of it are being improperly used or reported on, it can skew the true representation.
Data cleaning may solve some of your immediate data quality issues, but it won’t prevent new ones from cropping up down the road. Data quality is about more than existing data, it’s also about the processes you use to create the data, how data is stored, where data is used, who can use it, and how it can be altered. That’s why you need to approach data quality with a broader scope than data cleaning alone can provide. Multiple data quality issues exist simultaneously, and they must ALL be addressed to solve the problem.
To an extent, yes, they help. However, much like data cleaning, a data warehouse should not act as a catch-all for your quality issues.
Why?
Data is coming into the data warehouse from all over the place. You have people relying on systems like CRM and ERP to source data for the warehouse. You may also have people populating the data warehouse with information sourced from a data mart or data cube. Data silos between these systems means you could easily have multiple versions of the same data existing between them. The data warehouse, therefore, cannot exist as the single source of truth.
Again, this point is part of a larger data quality umbrella. Inaccurate or incomplete data can be caused by data entry errors. Part of establishing a long-term data quality plan is having guidelines for how users can enter data. And that’s great!
Rules for data entry will help teams prevent issues, but those users need support, too. Established processes and data management technologies guide the human interaction and must also be considered when building a complete data quality approach. Data entry issues are only a fraction of the whole when it comes to data quality.
If we know the dangers of poor data quality, and we know the misconceptions around solving data quality, how do we move forward? What steps must we take to address each moving part? Here are some tips for improving data quality:
The simplest initial step to data quality is data cleaning and list matching. Data cleaning looks for inaccurate, incomplete, and irrelevant data to discard. It will peel off the first layer of data quality.
Aligned to data cleaning is list matching. Matching the data across and between sources will help you identify duplicates and recognize misrepresented data.
MDM supports the long-term goals of data quality by defining rules for how data can be created, organized, stored, used, and disposed of. Creating a Master Data Management plan requires time and forethought. To define the rules though, you must first analyze your data integrity.
Understanding the accuracy and consistency of your current data will help guide you going forward. Which data are you currently requiring? Where is the incomplete data? Should new fields be required? How old is your data? Is it all still relevant? A consultant can help you perform a proper data integrity assessment.
If you need a jumpstart to your MDM strategy, start by building a data dictionary. Data dictionaries are a less intense version of an MDM plan. They provide loose guidelines and insights about the data so users can grasp the basics. As you get started on your bigger MDM plan, having a data dictionary referenceable for your teams is a helpful intermediary.
One of the easiest ways to build a single source of truth and truly know how your data compares between systems is to integrate those systems together. Whether you’re connecting your ERP and CRM, ERP to eCommerce, Marketing Automation & CRM, or otherwise, integration does one very important thing to help data quality: it removes siloes.
Data is no longer restricted to one system and hidden from the view of other departments or teams. Sharing that information across the organization illuminates your data. It enables you to build workflows that not only join the data throughout your organization but help fill the communication and process gaps between divisions of your business. Your reporting also becomes more accurate. If you’re building reports based on the same data in separate systems, but getting different outcomes, that’s a clear indicator that your data quality may be off. Any time you can eliminate data siloes, you are helping the cause for data quality.
If you’re utilizing AI tools, or even a BI-focused CRM, you may already have some data augmentation capabilities available to you. AI is a popular tool for managing data quality, as a recent survey by O’Reilly found. Nearly half of surveyed respondents indicated they used AI, machine learning, or data analysis tools to address data quality.
Many software providers are now building data augmentation and AI into their solutions to enrich customer profiles and fill in the gaps on missing data. Not only do these tools help manage data quality, but they also simplify data entry to add some helpful data quality assistance.
Find out if any of your software providers offer data augmentation capabilities. If you do not have data augmentation available to you through your current software provider, consult with a professional on your next best option.
Data quality is too often overlooked, but it’s far too important to ignore. Every year, 25-30% of data becomes inaccurate, negatively impacting the capabilities of sales and marketing to create effective strategies. Identifying, cleaning, enriching, and protecting your data for the long-term will help create the ideal environment for intelligent reporting, analytics, and most importantly business growth.
Fill out the form below and we will contact you